The Role of Agriculture Datasets for Machine Learning in Modern Business
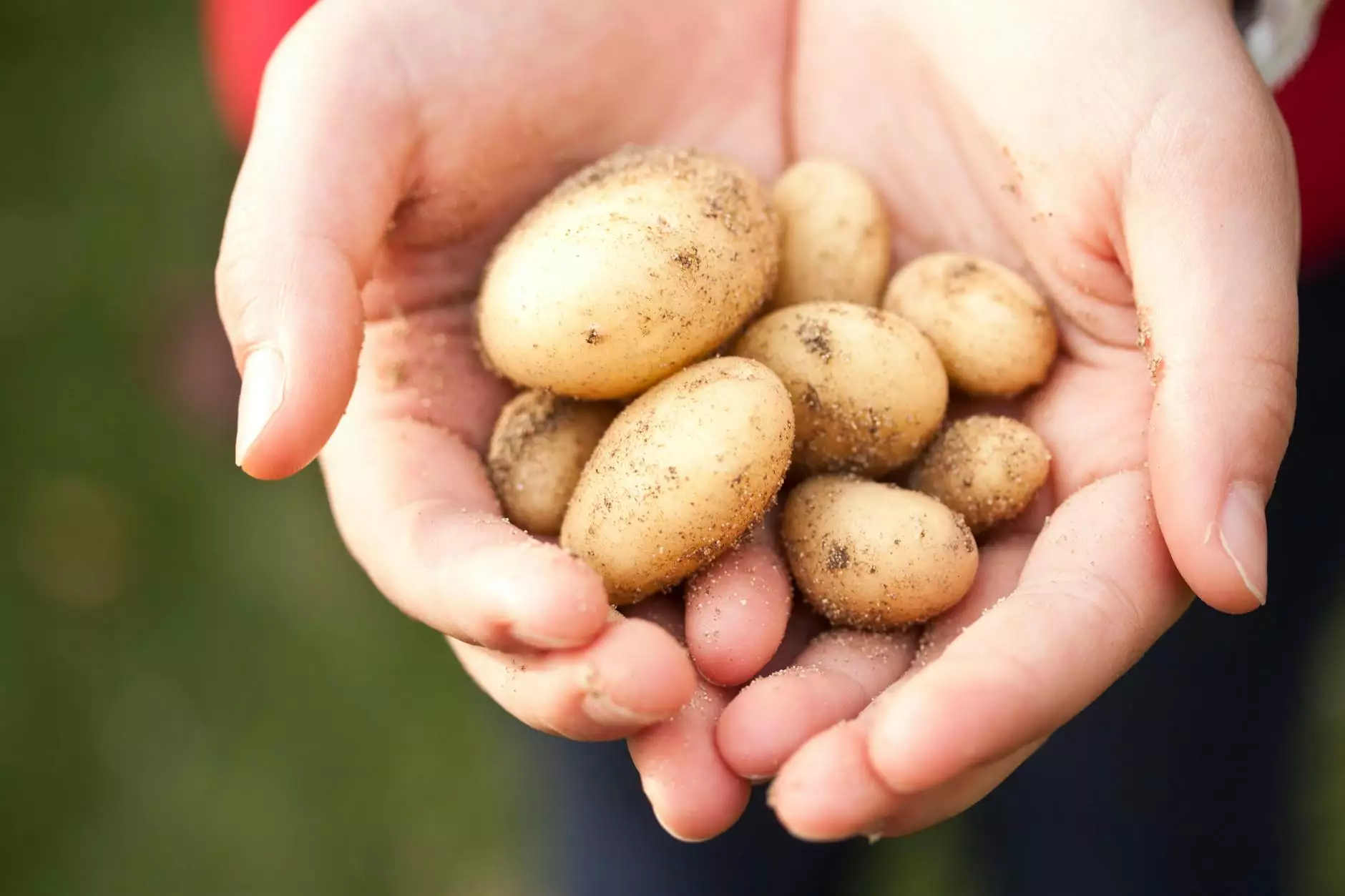
In the rapidly evolving landscape of technology, businesses across various sectors are increasingly recognizing the transformative power of data. Among the most intriguing applications is the utilization of agriculture datasets for machine learning, which empowers businesses to optimize their operations, enhance decision-making, and drive innovation. This article explores how such datasets can benefit various industries, particularly focusing on home services, keys, and locksmiths, while providing insights into leveraging machine learning for superior outcomes.
Understanding Agriculture Datasets for Machine Learning
Agriculture datasets are collections of data related to farming, crop yields, soil health, weather patterns, and market trends. These datasets are vital for machine learning algorithms, which need vast amounts of data to learn patterns, make predictions, and optimize processes. Machine learning is a subset of artificial intelligence that enables systems to improve from experience, and these datasets serve as the foundation for such advancements.
Why Agriculture Datasets Matter
The significance of agriculture datasets cannot be overstated, especially in the context of machine learning applications. Here are a few reasons why they are crucial:
- Enhanced Decision Making: By analyzing trends and patterns in agriculture data, businesses can make informed decisions that lead to increased profitability.
- Predictive Modeling: Machine learning models trained on agricultural data can predict future outcomes, aiding in supply chain management and inventory control.
- Resource Optimization: Understanding data related to resource allocation helps businesses minimize waste and allocate resources more effectively.
- Market Analysis: Analyzing agricultural datasets allows businesses to understand market demands and trends that can directly influence their operations.
Applications of Agriculture Datasets for Machine Learning in Business
Various sectors can benefit significantly from the integration of agriculture datasets for machine learning. Here, we discuss three main fields: home services, keys and locksmiths, and general business operations.
1. Home Services
For businesses in the home services sector, understanding customer needs is paramount. Using agriculture datasets combined with machine learning can lead to more customized services. Numerous applications include:
- Pest Control: Businesses can utilize data regarding pest populations, their biological cycles, and environmental conditions to tailor pest control services effectively according to seasonal trends.
- Landscape Maintenance: By analyzing soil and hydration data, home service providers can optimize landscaping solutions and maintenance schedules, ensuring that plants thrive according to their specific needs.
- Yield Prediction: Home service businesses that are also engaged in home gardens can use agricultural datasets to predict crop yields, therefore advising clients on the best planting schedules.
2. Keys & Locksmiths
For locksmiths and key service providers, the integration of machine learning can streamline operations significantly:
- Security Analytics: By applying agricultural data methodologies, locksmiths can analyze neighborhood crime rates, helping them advise clients on the best security measures based on agrarian insights.
- Demand Forecasting: Machine learning algorithms can leverage historical data from agricultural trends to predict periods of high demand for lock installations or repairs.
- Customer Insights: Analyzing data can help locksmiths understand which services are more commonly requested in specific environments, allowing them to tailor marketing strategies accordingly.
3. General Business Operations
The applications extend beyond specific business types. A plethora of operations can benefit from embracing agricultural datasets:
- Supply Chain Efficiency: Understanding the relationship between supply and demand through data can enhance operational efficiencies, particularly in managing inventory levels.
- Cost Reduction: Machine learning can reveal insights into unnecessary expenditures, recommending ways to cut costs while maintaining quality.
- Product Development: Businesses can analyze market trends related to agriculture to innovate new products that fulfill emerging customer needs.
Challenges in Leveraging Agriculture Datasets for Machine Learning
While the advantages are clear, there are challenges that businesses must navigate when implementing agriculture datasets for machine learning:
- Data Quality: The efficacy of machine learning heavily relies on high-quality datasets. Businesses must ensure that the data they use is accurate, comprehensive, and up-to-date.
- Technical Expertise: Implementing machine learning solutions requires technical prowess. Businesses may need to invest in training or hiring skilled professionals.
- Integration with Existing Systems: Adapting machine learning technologies to existing business operations can be a complex process, requiring careful planning and execution.
How to Get Started with Agriculture Datasets and Machine Learning
Successfully leveraging agriculture datasets for machine learning demands a strategic approach. Here’s how businesses can begin their journey:
1. Identify Objectives
Begin by clearly defining the goals your business aims to achieve through machine learning. Whether it’s improving customer service, optimizing efficiency, or predicting trends, a well-defined objective sets the foundation for successful implementation.
2. Collect and Clean Data
Gather relevant agricultural and operational data. Ensure that the data is cleaned and pre-processed to eliminate inconsistencies or errors. This step is crucial for building a robust machine learning model.
3. Choose the Right Tools
Select the appropriate machine learning tools and frameworks that suit your business requirements and technical capabilities. Popular choices include Python libraries like TensorFlow and scikit-learn.
4. Train Machine Learning Models
Utilize your cleaned datasets to train machine learning models. Ensure that these models are well-tested and validated against various scenarios to guarantee accuracy and reliability.
5. Monitor and Optimize
Post-deployment, continuously monitor the efficacy of your machine learning models. Analyzing their performance will guide necessary adjustments, ensuring that the systems evolve to adapt to new challenges and opportunities.
Conclusion
The landscape of business is being reshaped by the integration of agriculture datasets for machine learning. From enhancing efficiency in home services to providing integral data for locksmith operations, the applications are extensive and beneficial. By harnessing the potential of these datasets, businesses can drive growth, optimize resources, and stay ahead of the competition.
The journey toward implementing machine learning is filled with challenges, but the long-term benefits in decision-making, customer satisfaction, and operational efficiency are undeniable. As we step into a data-driven future, embracing the power of agriculture datasets may just be the secret to unlocking your business's full potential.
agriculture dataset for machine learning